Multi-Sensor and Multi-Temporal Remote Sensing
ebook ∣ Specific Single Class Mapping
By Anil Kumar
Sign up to save your library
With an OverDrive account, you can save your favorite libraries for at-a-glance information about availability. Find out more about OverDrive accounts.
Find this title in Libby, the library reading app by OverDrive.
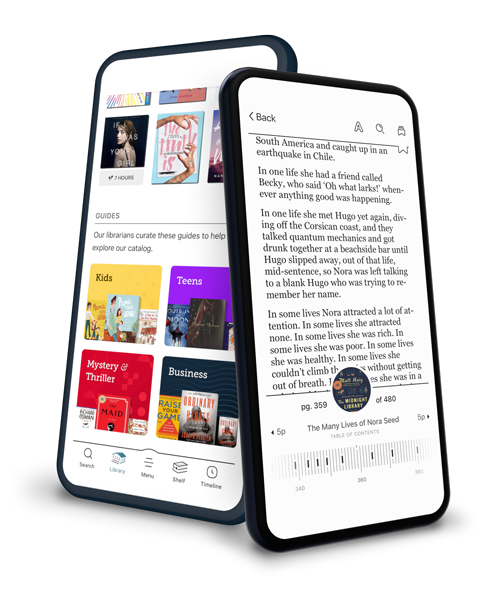
Search for a digital library with this title
Title found at these libraries:
Library Name | Distance |
---|---|
Loading... |
This book elaborates fuzzy machine and deep learning models for single class mapping from multi-sensor, multi-temporal remote sensing images while handling mixed pixels and noise. It also covers the ways of pre-processing and spectral dimensionality reduction of temporal data. Further, it discusses the 'individual sample as mean' training approach to handle heterogeneity within a class. The appendix section of the book includes case studies such as mapping crop type, forest species, and stubble burnt paddy fields.
Key features:
This book is intended for graduate/postgraduate students, research scholars, and professionals working in environmental, geography, computer sciences, remote sensing, geoinformatics, forestry, agriculture, post-disaster, urban transition studies, and other related areas.