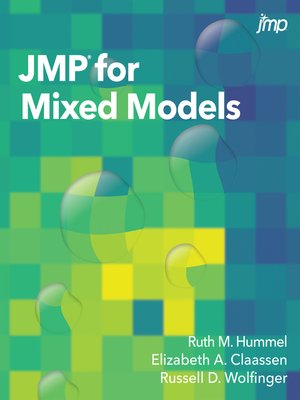
Sign up to save your library
With an OverDrive account, you can save your favorite libraries for at-a-glance information about availability. Find out more about OverDrive accounts.
Find this title in Libby, the library reading app by OverDrive.
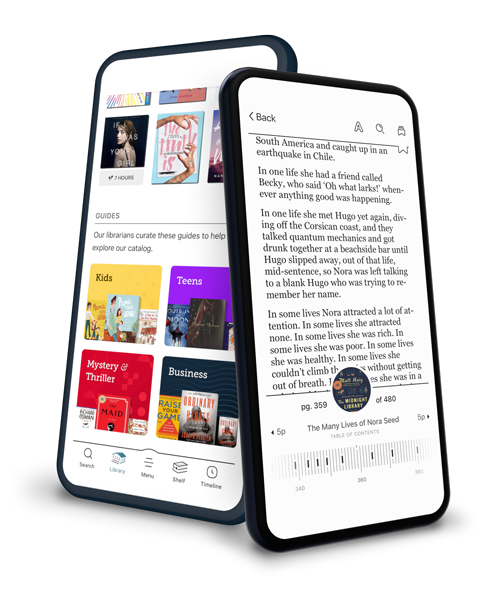
Search for a digital library with this title
Title found at these libraries:
Library Name | Distance |
---|---|
Loading... |
Discover the power of mixed models with JMP and JMP Pro.
Mixed models are now the mainstream method of choice for analyzing experimental data. Why? They are arguably the most straightforward and powerful way to handle correlated observations in designed experiments. Reaching well beyond standard linear models, mixed models enable you to make accurate and precise inferences about your experiments and to gain deeper understanding of sources of signal and noise in the system under study. Well-formed fixed and random effects generalize well and help you make the best data-driven decisions.
JMP for Mixed Models brings together two of the strongest traditions in SAS software: mixed models and JMP. JMP's groundbreaking philosophy of tight integration of statistics with dynamic graphics is an ideal milieu within which to learn and apply mixed models, also known as hierarchical linear or multilevel models. If you are a scientist or engineer, the methods described herein can revolutionize how you analyze experimental data without the need to write code.
Inside you'll find a rich collection of examples and a step-by-step approach to mixed model mastery. Topics include: