An Introduction to Lifted Probabilistic Inference
ebook ∣ Neural Information Processing series
By Guy Van den Broeck
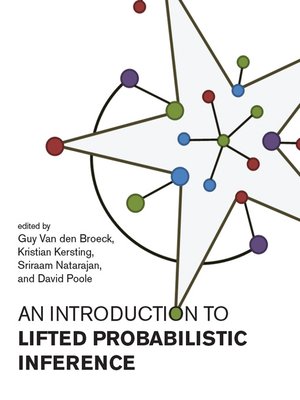
Sign up to save your library
With an OverDrive account, you can save your favorite libraries for at-a-glance information about availability. Find out more about OverDrive accounts.
Find this title in Libby, the library reading app by OverDrive.
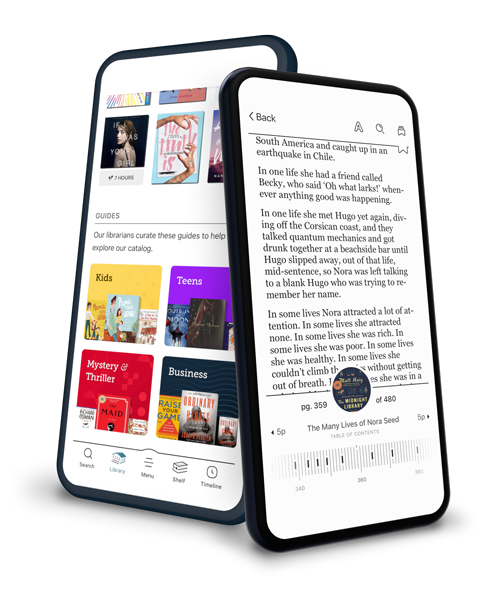
Search for a digital library with this title
Title found at these libraries:
Library Name | Distance |
---|---|
Loading... |
Recent advances in the area of lifted inference, which exploits the structure inherent in relational probabilistic models.
Statistical relational AI (StaRAI) studies the integration of reasoning under uncertainty with reasoning about individuals and relations. The representations used are often called relational probabilistic models. Lifted inference is about how to exploit the structure inherent in relational probabilistic models, either in the way they are expressed or by extracting structure from observations. This book covers recent significant advances in the area of lifted inference, providing a unifying introduction to this very active field.
After providing necessary background on probabilistic graphical models, relational probabilistic models, and learning inside these models, the book turns to lifted inference, first covering exact inference and then approximate inference. In addition, the book considers the theory of liftability and acting in relational domains, which allows the connection of learning and reasoning in relational domains.
Statistical relational AI (StaRAI) studies the integration of reasoning under uncertainty with reasoning about individuals and relations. The representations used are often called relational probabilistic models. Lifted inference is about how to exploit the structure inherent in relational probabilistic models, either in the way they are expressed or by extracting structure from observations. This book covers recent significant advances in the area of lifted inference, providing a unifying introduction to this very active field.
After providing necessary background on probabilistic graphical models, relational probabilistic models, and learning inside these models, the book turns to lifted inference, first covering exact inference and then approximate inference. In addition, the book considers the theory of liftability and acting in relational domains, which allows the connection of learning and reasoning in relational domains.