Comparison of different features sets and classifiers for emotion recognition of speech
ebook ∣ Vergleich von verschiedenen Merkmalssätzen und Klassifizierern zur Emotionserkennung von Sprache · Aus der Reihe: e-fellows.net stipendiaten-wissen
By Tobias Gruber
Sign up to save your library
With an OverDrive account, you can save your favorite libraries for at-a-glance information about availability. Find out more about OverDrive accounts.
Find this title in Libby, the library reading app by OverDrive.
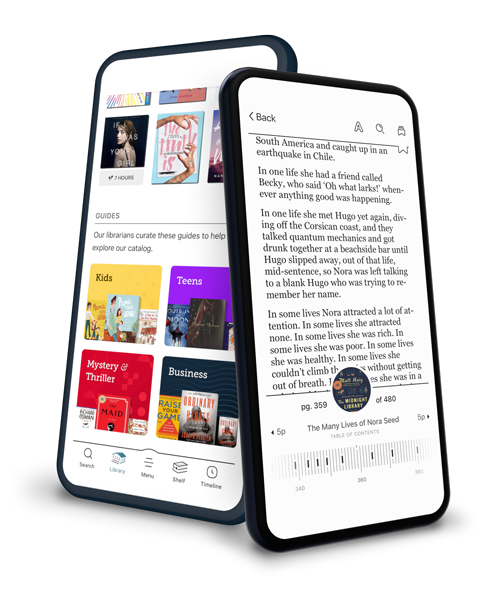
Search for a digital library with this title
Title found at these libraries:
Library Name | Distance |
---|---|
Loading... |
Bachelor Thesis from the year 2014 in the subject Electrotechnology, grade: 1,0, University of Stuttgart (Institut für Signalverarbeitung und Systemtheorie), course: Elektrotechnik und Informationstechnik, language: English, abstract: This thesis deals with emotion recognition from speech signals using several feature
sets and classifiers. Feature sets with different sizes are compared: the feature set
of the Institute for Signal Processing and System Theory as well as standardised
feature sets of eight paralinguistic challenges. The question is whether there is a
connection between the size of a feature set and the performance. The feature sets
are investigated with SFFS and without in combination with Naive Bayes classifier,
k-Nearest-Neighbour classifier and Support Vector Machine. The goal of this thesis
is to find those features which are selected most commonly for good performance. Diese Arbeit befasst sich mit der Erkennung von Emotionen aus Sprachsignalen. Es werden verschiedene Merkmalsätze und Klassifizierer auf ihre Leistungsfähigkeit getestet. Dabei werden Merkmalsätze mit unterschiedlichen Größen verglichen: der Merkmalsatz vom Institut für Signalverarbeitung und Systemtheorie sowie standardisierte Merkmalsätze von acht Wettbewerben, in denen paralinguistische Informationen erkannt werden sollten. Die Frage
ist, ob es einen Zusammenhang zwischen der Größe eines Merkmalsatzes und der Leistungsfähigkeit gibt. Die Merkmalsätze werden sowie mit auch als ohne Merkmalsauswahl (SFFS) in Kombination mit dem Naiven Bayes Klassifizierer, k-Nächste-Nachbarn Klassifizierer und einer Support Vector Machine untersucht. Das Ziel dieser Arbeit ist, die Merkmale zu finden, die bei den besten Merkmalsätzen am häufigsten ausgewählt wurden.