Retrieval-Augmented Generation (RAG) using Large Language Models
audiobook (Unabridged)
By Anand Vemula
Sign up to save your library
With an OverDrive account, you can save your favorite libraries for at-a-glance information about availability. Find out more about OverDrive accounts.
Find this title in Libby, the library reading app by OverDrive.
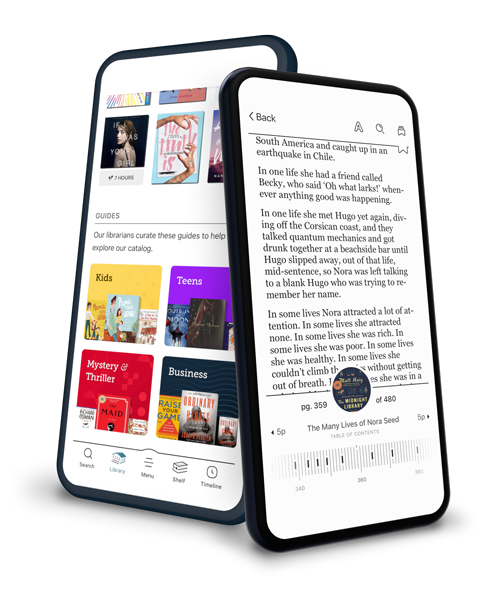
Search for a digital library with this title
Title found at these libraries:
Loading... |
This audiobook is narrated by a digital voice.
Unlocking Knowledge" explores the transformative potential of Retrieval-Augmented Generation (RAG) using Large Language Models (LLMs). In this comprehensive guide, readers embark on a journey through the intersection of cutting-edge natural language processing techniques and innovative information retrieval strategies.
The book begins by elucidating the fundamental concepts underlying RAG, delineating its evolution and significance in contemporary AI research. It elucidates the symbiotic relationship between retrieval-based and generation-based models, showcasing how RAG seamlessly integrates these methodologies to produce contextually enriched responses.
Through detailed explanations and practical insights, "Unlocking Knowledge" guides readers through the implementation process of RAG, from setting up the computational environment to fine-tuning model parameters. It navigates the complexities of data collection and preprocessing, emphasizing the importance of dataset quality and relevance.
Readers delve into the intricacies of training the retriever and generator components, learning strategies to optimize model performance and mitigate common challenges. The book illuminates evaluation metrics for assessing RAG systems, offering guidance on iterative refinement and optimization.