Understanding Large Language Models
audiobook (Unabridged) ∣ : A Guide to Transformer Architectures and NLP Applications
By Anand Vemula
Sign up to save your library
With an OverDrive account, you can save your favorite libraries for at-a-glance information about availability. Find out more about OverDrive accounts.
Find this title in Libby, the library reading app by OverDrive.
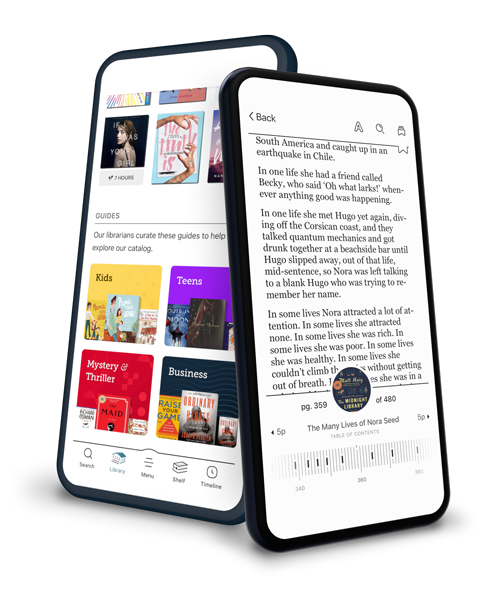
Search for a digital library with this title
Title found at these libraries:
Loading... |
This audiobook is narrated by a digital voice.
In the ever-evolving world of language processing, "Understanding Large Language Models" offers a comprehensive guidebook. It delves into the inner workings of both Large Language Models (LLMs) and the revolutionary Transformer architectures that power them.
The book begins by establishing the foundation. Part 1 introduces Natural Language Processing (NLP) and the challenges it tackles. It then unveils LLMs, exploring their capabilities and the impact they have on various industries. Ethical considerations and limitations of these powerful tools are also addressed.
Part 2 equips you with the necessary background. It dives into the essentials of Deep Learning for NLP, explaining Recurrent Neural Networks (RNNs) and their shortcomings. Traditional NLP techniques like word embeddings and language modeling are also explored, providing context for the advancements brought by transformers.
Part 3 marks the turning point. Here, the book unveils the Transformer architecture, the engine driving LLMs. You'll grasp its core principles, including the encoder-decoder structure and the critical concept of attention, which allows the model to understand relationships within text. The chapter delves into the benefits transformers offer, such as speed, accuracy, and their ability to capture long-range dependencies in language.
Part 4 bridges the gap between theory and practice. It explores the data preparation process for training LLMs and the challenges associated with handling massive datasets. Optimization techniques for efficient learning are explained, along with the concept of fine-tuning pre-trained LLMs for specific applications.